Course – Lesson 4: III. Testing a Hypothesis
LESSON 4:III – Q+A WITH AUTHORS KATHERINE ORTEGA COURTNEY, PHD AND DOMINIC CAPPELLO
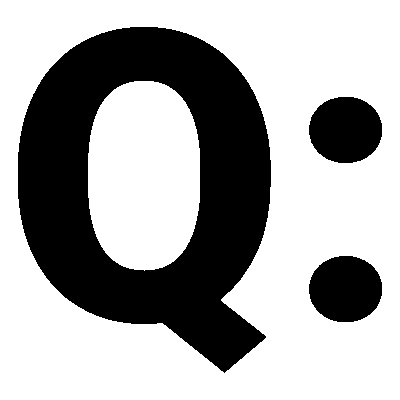
What is "fidelity" in terms of our team's innovation, and why is it important?
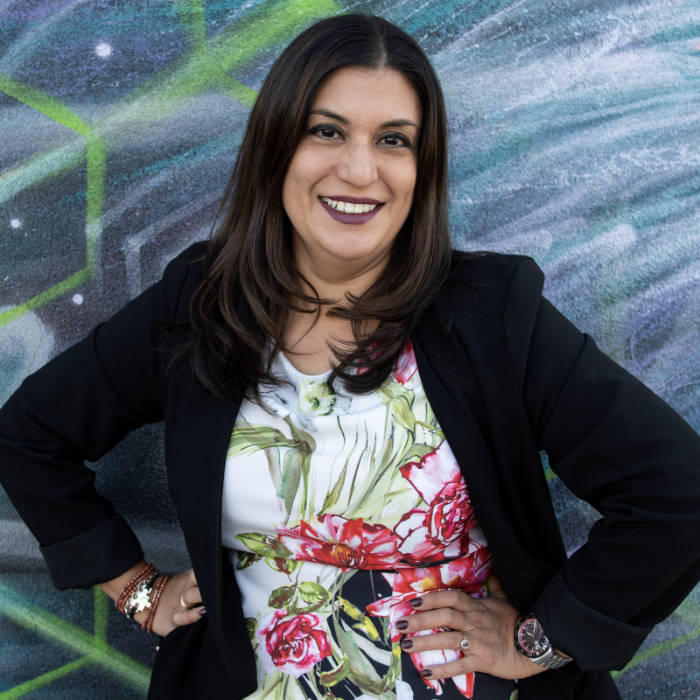
Katherine Ortega Courtney, PhD responds:
In the planning phase we designed the innovation or initiative. In the action phase we wish to follow that plan in all its details. For example, let’s say a school district has a new training for all school staff to help them identify students who might be experiencing trauma associated with ACEs (Adverse Childhood Experiences.) The training is four days with half the time spent on coaching. The evaluation of the training showed high marks from participants in terms of increased knowledge. A second school district decides to do the training but reduces it to two days and eliminates the coaching aspect. They have changed the training significantly and fidelity is not being respected. The second school district may get very different outcomes (knowledge increase among participants may be lower) as a result of changing the intervention).
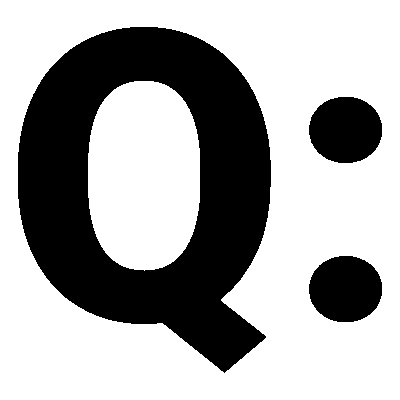
What types and means of data collection are useful to increase fidelity?
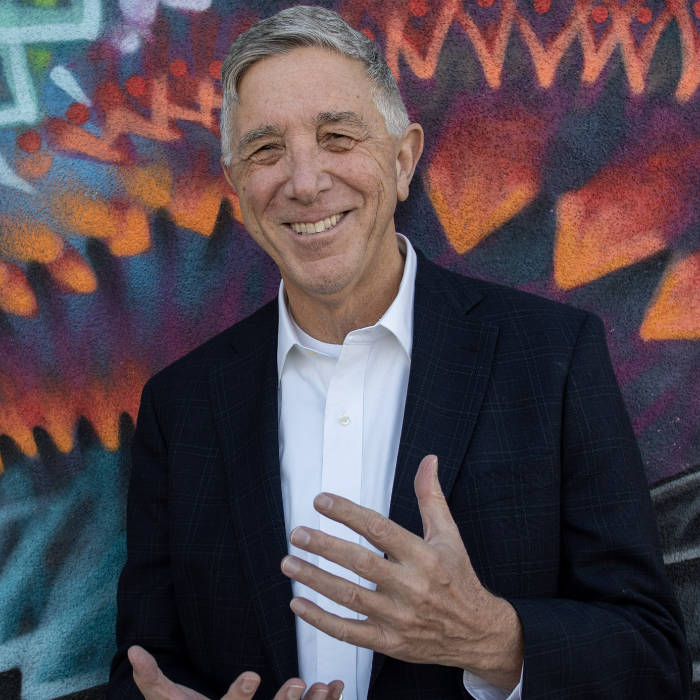
Dominic Cappello responds:
The first step towards increasing fidelity with an experiment is to ensure that those responsible for its implementation are on the same page. This might be accomplished through a training or team meeting, and followed up with a short survey to identify any potential inconsistencies in how the specific elements of the experiment are understood.
Second, it is helpful to track what experimental activities are occurring at the individual or case levels, depending on the experiment. This might be done with the use of a spreadsheet (such as in Microsoft Excel or Google Sheets); a paper tracking form; or even an online survey tool like Survey Monkey.
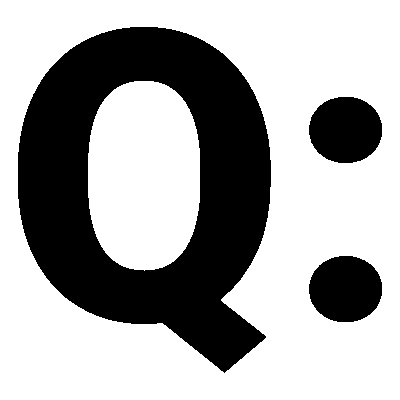
How do we establish a baseline prior to implementing our innovation?
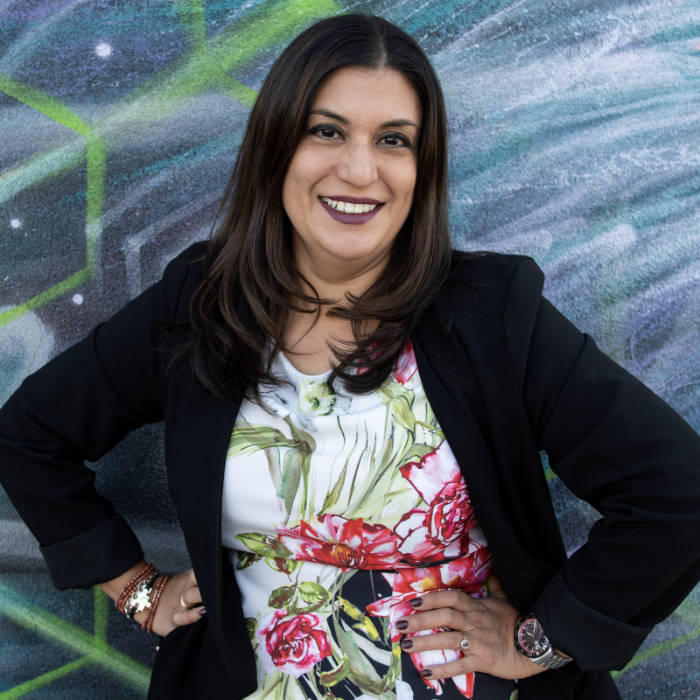
Katherine Ortega Courtney, PhD responds:
Baseline data is necessary to gauge what, if any, changes occur as a result of your experiment. Baseline data related to your experiment area may be readily available to you prior to implementing your activities; however, some experiments may address challenges for which no applicable baseline data exists, and that data must be collected. A good array of baseline data is likely to consist of both.
The Resilient Community Experience Survey will serve as great baseline data. If this survey is implemented at the beginning of the 100% New Mexico initiative, before any innovations have taken place, that can serve as a baseline. If it is implemented again after action teams have begun implementing innovations, it could also serve as a great way to measure change.
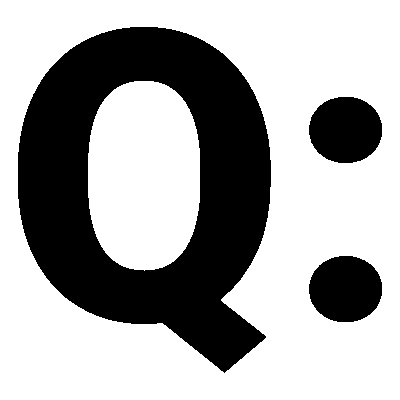
How would the concept of a "control group" apply to our local experiment?
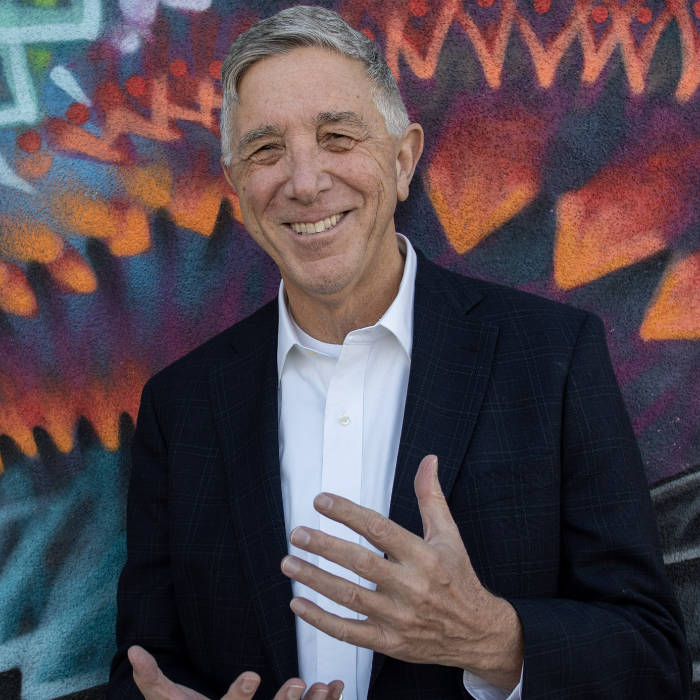
Dominic Cappello responds:
In the world of science, experiments are considered “controlled” when there is, in addition to a population receiving the treatment or intervention, a similar group who does not receive it — the control group. This way, the magnitude of any changes noted in the “experimental group” can be accounted for through comparison with the control group.
Carefully designed experiments will usually assign similar clients or cases at random to either the experimental group or the control group (thus making the experiment not only controlled, but “randomized”). However, in the field (the “real world”) this isn’t always possible or practical. In some situations, clients will self-select to be part of one or the other.
How does my team anticipate what data we will need for evaluation?
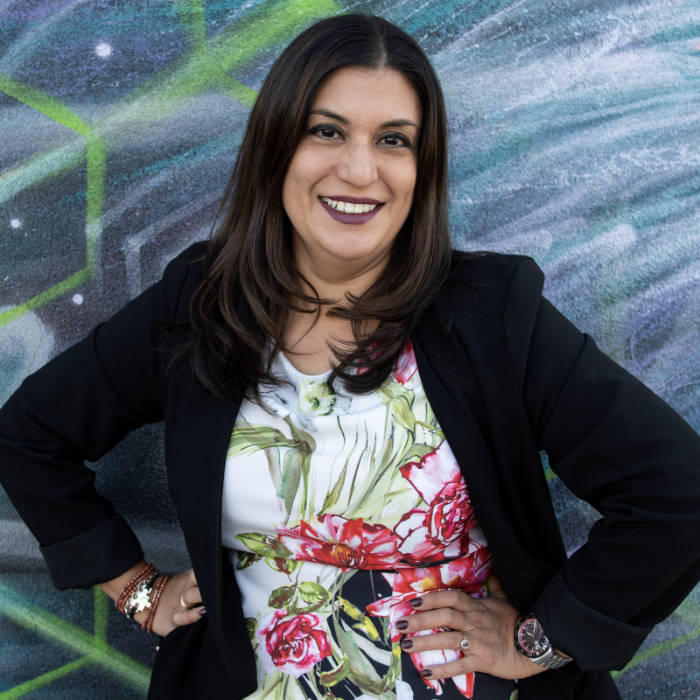
Katherine Ortega Courtney, PhD responds:
Data for evaluation will need to include: data that are aligned with any baseline data collected (For example, results of the initial Resilient Community Experience Survey). Another example would be pre- and post-survey responses to a particular intervention such as a training for law enforcement on trauma sensitive practices); data that track fidelity (For example, data related to possible alternative explanations (things that could have an effect on outcomes but are not related to the actual experiment or intervention in question).
What are other effects of implementing a new practice that needs to be evaluated?
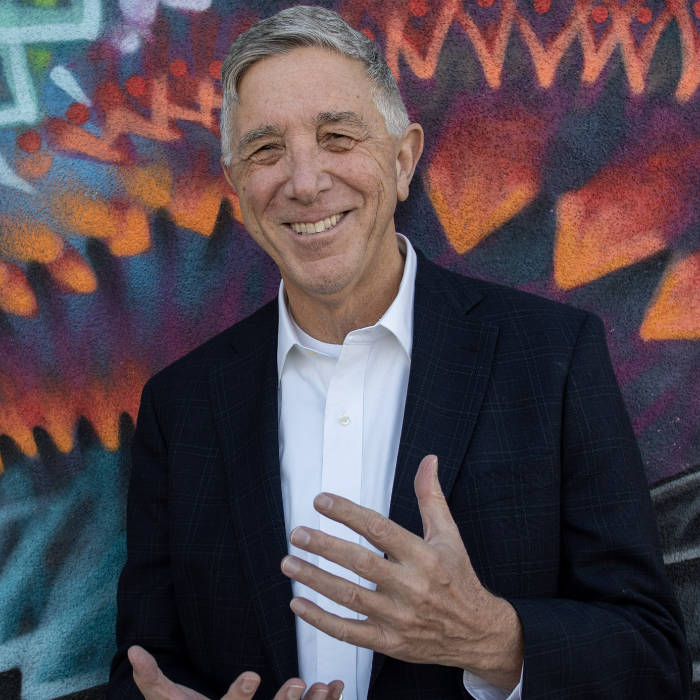
Dominic Cappello responds:
Our 100% New Mexico initiative will be testing many new innovative ways of increasing service quality and accessibility. The implementation and evaluation of any new program, practice or policy should also take into account the circumstances and secondary effects of implementing a new practice. When evaluating an experiment, it is important to consider not only whether the activities themselves are effective towards their goal, but: whether they are sustainable; what effects they have on staff morale; whether they require additional resources; and if they are applicable to address other challenges.